Abstract¶
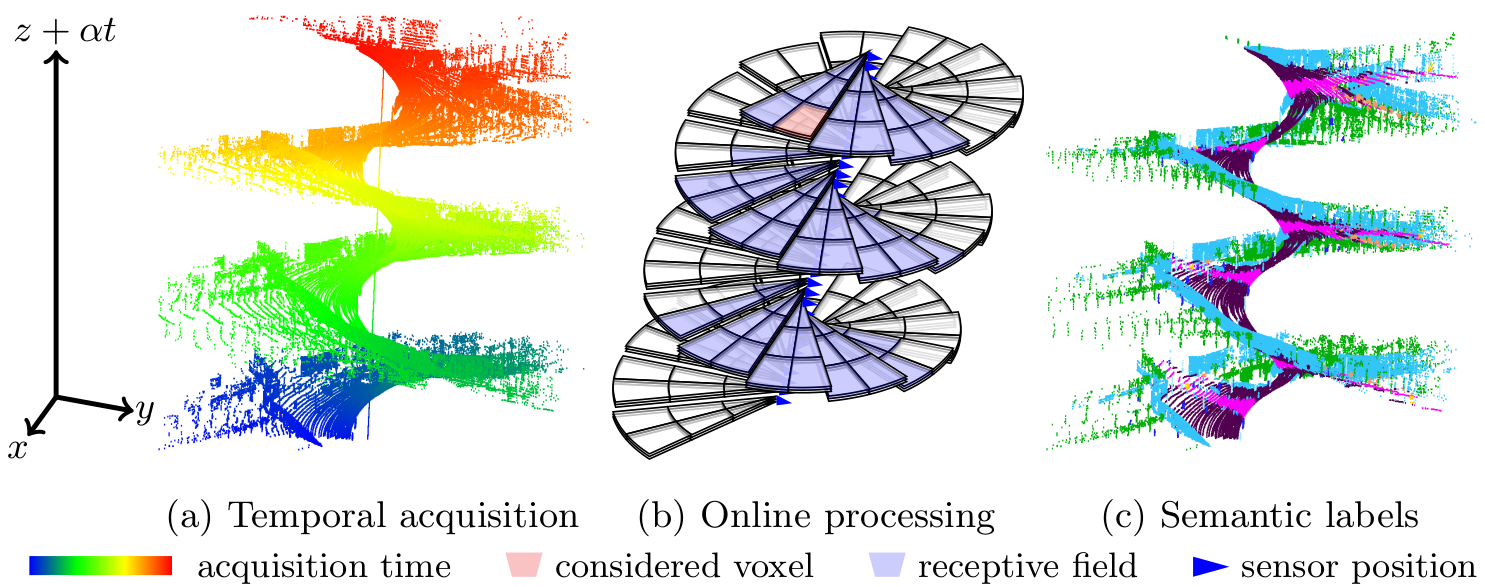
Real-time processing of LiDAR sequences is critical for autonomous driving. However, most semantic segmentation datasets and algorithms split this data into frames covering a full rotation of the sensor. This leads to an acquisition latency which is not realistic for real-time semantic segmentation. We address this issue with two key contributions. First, we introduce HelixNet, a 10-billion point dataset with fine-grained timestamps and sensor rotation information, which allows us to precisely assess the real-time readiness of LiDAR segmentation methods. Second, we propose Helix4D, a compact and efficient spatio-temporal transformer architecture specifically designed for rotating LiDAR sequences. Helix4D reaches accuracy on par with the best segmentation algorithms with a reduction of over 5× in terms of latency and 50× in model size.
Video¶
Citation¶
@article{loiseau22online,
title={Online Segmentation of LiDAR Sequences: Dataset and Algorithm.},
author={Romain Loiseau and Mathieu Aubry and Loic Landrieu},
journal={ECCV},
year={2022}
}
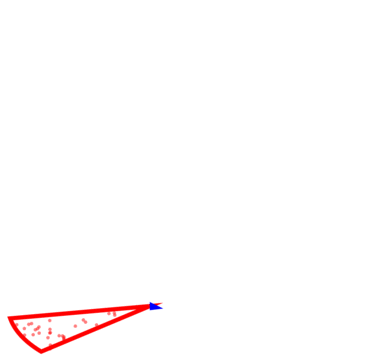
Acknowledgements¶
This work was supported in part by ANR project READY3D ANR-19-CE23-0007 and was granted access to the HPC resources of IDRIS under the allocation 2022-AD011012096R1 made by GENCI. The point cloud sequence of HelixNet was acquired during the Stereopolis II project (N. Paparoditis et al. "Stereopolis II: A multi-purpose and multi-sensor 3D mobile mapping system for street visualisation and 3D metrology." Revue française de photogrammétrie et de télédétection, 2012). HelixNet was annotated by FUTURMAP. We thank Zenodo for hosting the dataset. We thank François Darmon, Tom Monnier, Mathis Petrovich and Damien Robert for inspiring discussions and valuable feedback.