Abstract¶
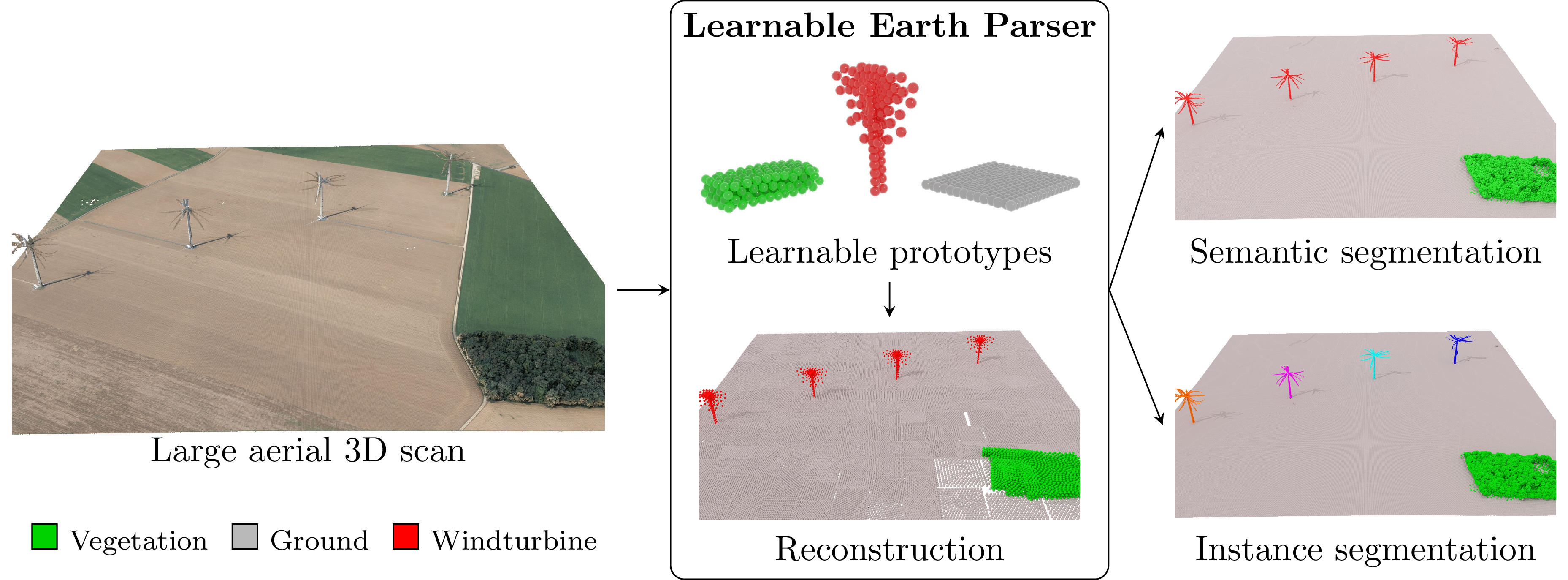
We propose an unsupervised method for parsing large 3D scans of real-world scenes with easily-interpretable shapes. This work aims to provide a practical tool for analyzing 3D scenes in the context of aerial surveying and mapping, without the need for user annotations. Our approach is based on a probabilistic reconstruction model that decomposes an input 3D point cloud into a small set of learned prototypical 3D shapes. The resulting reconstruction is visually interpretable and can be used to perform unsupervised instance and low-shot semantic segmentation of complex scenes. We demonstrate the usefulness of our model on a novel dataset of seven large aerial LiDAR scans from diverse real-world scenarios. Our approach outperforms state-of-the-art unsupervised methods in terms of decomposition accuracy while remaining visually interpretable.
The Earth Parser Dataset - A new dataset to train and evaluate parsing methods on large, uncurated aerial LiDAR scans¶
Learnable Earth Parser - An unsupervised method for parsing large 3D scans of real-world scenes into interpretable parts¶
Video¶
Citation¶
If you find this project useful for your research, please cite:
@article{loiseau2024learnable,
title={Learnable Earth Parser: Discovering 3D Prototypes in Aerial Scans},
author={Romain Loiseau and Elliot Vincent and Mathieu Aubry and Loic Landrieu},
journal={CVPR},
year={2024}
}
Acknowledgements¶
This work was supported in part by ANR project READY3D ANR-19-CE23-0007, ANR under the France 2030 program under the reference ANR-23-PEIA-0008, and was granted access to the HPC resources of IDRIS under the allocation 2022-AD011012096R2 made by GENCI. The work of MA was partly supported by the European Research Council (ERC project DISCOVER, number 101076028). The scenes of Earth Parser Dataset were acquired and annotated by the LiDAR-HD project. We thank Zenodo for hosting the dataset. We thank Zeynep Sonat Baltaci, Emile Blettery, Nicolas Dufour, Antoine Guedon, Helen Mair Rawsthorne, Tom Monnier, Damien Robert, Mathis Petrovich and Yannis Siglidis for inspiring discussions and valuable feedback.